Background
Personalized (N-of-1) trials are patient-centered experiments designed to identify the optimal personalized treatment for each individual patient. The methodology is useful in situations involving evidence for heterogeneity of treatment effects (HTE).
Cheung-Mitsumoto index (CMI) indicates the potential utility of personalized (N-of-1) trials by providing a measure of the extent of HTE. Traditionally, HTE is estimated by the standard deviation of random treatment effects ('sigmaB') in a mixed effects model. The CMI measures HTE by intepreting the strength of 'sigmaB' relative to the between-subject variation, within-subject variation, as well as the population-level average treatment effect.
Interpretation of CMI: The CMI takes on values between 0 and 1. A high value (close to 1) indicates N-of-1 trials are useful; a low value indicates otherwise. Under special settings of N-of-1 trials described in Cheung & Mitsumoto (2022), the CMI is equal to the statistical power of declaring N-of-1 trials are superior to non-N-of-1 trial alternative. Thus, as a rule of thumb, CMI > 0.8 indicates high feasibility of N-of-1 trials.
Reference: Cheung, K. & Mitsumoto, H. (2022). Evaluating personalized (N-of-1) trials in rare diseases: how much experimentation is enough? Harvard Data Science Review, https://doi.org/10.1162/99608f92.e11adff0
Details of the calculator:
The CMI formula, given below, depends on 4 input parameters:
1. Between-subject standard deviation ('sigmaA'): In a mixed effect model, it is often the SD of the random intercepts.
2. Within-subject standard deviation ('sigma'): In a mixed effect model, it is often the SD of the noise distribution.
3. Standard deviation of treatment effects ('sigmaB'): In a mixed effect model, it is often the SD of the random treatment slopes.
4. Average treatment effect ('muB'): In a mixed effect model, it is often the coefficient of the fixed treatment effect.
Formula:
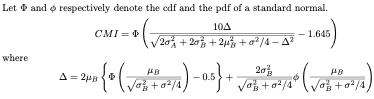